Vision Tutorial for iOS: What’s New With Face Detection?
Learn what’s new with Face Detection and how the latest additions to Vision framework can help you achieve better results in image segmentation and analysis. By Tom Elliott.
Sign up/Sign in
With a free Kodeco account you can download source code, track your progress, bookmark, personalise your learner profile and more!
Create accountAlready a member of Kodeco? Sign in
Sign up/Sign in
With a free Kodeco account you can download source code, track your progress, bookmark, personalise your learner profile and more!
Create accountAlready a member of Kodeco? Sign in
Contents
Vision Tutorial for iOS: What’s New With Face Detection?
35 mins
- Getting Started
- A Tour of the App
- Reviewing the Vision Framework
- Looking Forward
- Processing Faces
- Debug those Faces
- Selecting a Size
- Detecting Differences
- Masking Mayhem
- Assuring Quality
- Handling Quality Result
- Detecting Quality
- Offering Helpful Hints
- Segmenting Sapiens
- Using Metal
- Building Better Backgrounds
- Handling the Segmentation Request Result
- Removing the Background
- Saving the Picture
- Saving to Camera Roll
- Where to Go From Here?
Taking passport photos is a pain. There are so many rules to follow, and it can be hard to know if your photo is going to be acceptable or not. Luckily, you live in the 21st century! Say goodbye to the funny kiosks, and take control of your passport photo experience by using face detection from the Vision framework. Know if your photo will be acceptable before sending it off to the Passport Office!
In this tutorial you will:
- Learn how to detect roll, pitch and yaw of faces.
- Add quality calculation to face detection.
- Use person segmentation to mask an image.
Let’s get started!
Getting Started
Download the starter project by clicking the Download Materials button at the top or bottom of the tutorial.
The materials contain a project called PassportPhotos. In this tutorial, you’ll build a simple photo-taking app that will only allow the user to take a photo when the resulting image would be valid for a passport photo. The validity rules you’ll be following are for a UK passport photo, however it would be easy to replicate for any other country.
Open the starter project, select your phone from the available run targets and build and run.
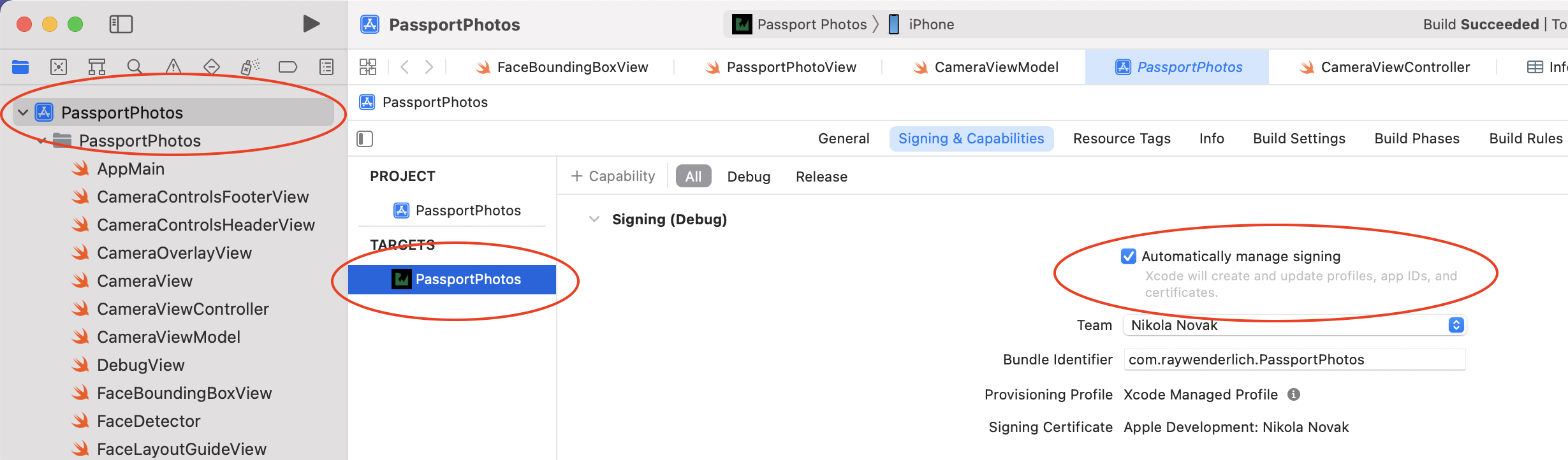
The app displays a front-facing camera view. A red rectangle and green oval are overlaid in the center of the screen. A banner across the top contains instructions. The bottom banner contains controls.
The center button is a shutter release for taking photos. On the left, the top button toggles the background, and the bottom — represented by a ladybug — toggles a debug view on and off. The button to the right of the shutter is a placeholder that gets replaced with a thumbnail of the last photo taken.
Bring the phone up to your face. A yellow bounding box starts tracking your face. Some face detection is already happening!
A Tour of the App
Let’s take a tour of the app now to get you oriented.
In Xcode, open PassportPhotosAppView.swift. This is the root view for the application. It contains a stack of views. A CameraView is at the bottom. Then, a LayoutGuide view (that draws the green oval on the screen) and optionally a DebugView. Finally, a CameraOverlayView is on top.
There are some other files in the app. Most of them are simple views used for various parts of the UI. In this tutorial, you will mainly update three classes: CameraViewModel
, the CameraViewController
UIKit view controller and FaceDetector
.
Open CameraViewModel.swift. This class controls the state for the entire app. It defines some published properties that views in the app can subscribe to. Views can update the state of the app by calling the single public method – perform(action:)
.
Next, open CameraView.swift. This is a simple SwiftUI UIViewControllerRepresentable
struct. It instantiates a CameraViewController
with a FaceDetector
.
Now open CameraViewController.swift. CameraViewController
configures and controls the AV capture session. This draws the pixels from the camera on to the screen. In viewDidLoad()
, the delegate of the face detector object is set. It then configures and starts the AV capture session. configureCaptureSession()
performs most of the setup. This is all basic setup code that you have hopefully seen before.
This class also contains some methods related to setting up Metal. Don’t worry about this just yet.
Finally, open FaceDetector.swift. This utility class has a single purpose — to be the delegate for the AVCaptureVideoDataOutput
setup in the CameraViewController
. This is where the face detection magic happens. :] More on this below.
Feel free to nose around the rest of the app. :]
Reviewing the Vision Framework
The Vision framework has been around since iOS 11. It provides functionality to perform a variety of computer vision algorithms on images and video. For example, face landmark detection, text detection, barcode recognition and others.
Before iOS 15, the Vision framework allowed you to query the roll and yaw of detected faces. It also provided the positions of certain landmarks like eyes, ears and nose. An example of this is already implemented in the app.
Open FaceDetector.swift and find captureOutput(_:didOutput:from:)
. In this method, the face detector sets up a VNDetectFaceRectanglesRequest
on the image buffer provided by the AVCaptureSession
.
When face rectangles are detected, the completion handler, detectedFaceRectangles(request:error:)
, is called. This method pulls the bounding box of the face from the face observation results and performs the faceObservationDetected
action on the CameraViewModel
.
Looking Forward
It’s time to add your first bit of code!
Passport regulations require people to look straight at the camera. Time to add this functionality. Open CameraViewModel.swift.
Find the FaceGeometryModel
struct definition. Update the struct by adding the following new properties:
let roll: NSNumber
let pitch: NSNumber
let yaw: NSNumber
This change allows you to store the roll, pitch and yaw values detected in the face in the view model.
At the top of the class, under the hasDetectedValidFace
property, add the following new published properties:
@Published private(set) var isAcceptableRoll: Bool {
didSet {
calculateDetectedFaceValidity()
}
}
@Published private(set) var isAcceptablePitch: Bool {
didSet {
calculateDetectedFaceValidity()
}
}
@Published private(set) var isAcceptableYaw: Bool {
didSet {
calculateDetectedFaceValidity()
}
}
This adds three new properties to store whether the roll, pitch and yaw of a detected face are acceptable for a passport photo. When each one updates, it will call the calculateDetectedFaceValidity()
method.
Next, add the following to the bottom of init()
:
isAcceptableRoll = false
isAcceptablePitch = false
isAcceptableYaw = false
This simply sets the initial values of the properties you just added.
Now, find the invalidateFaceGeometryState()
method. It’s a stub currently. Add the following code into that function:
isAcceptableRoll = false
isAcceptablePitch = false
isAcceptableYaw = false
Because no face is detected, you set the acceptable roll, pitch and yaw values to false
.